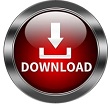

lowercase boolean: whether to convert strings to lowercase.Valid policy values are: “all”, “pretty_all”, “first”, “pretty_first”. removalPolicy: parameter to remove patterns from text with a given policy.replacement: replacement string to apply when regexes match.Default is “]*>” (e.g., it removes all HTML tags). cleanUpPatterns: normalization regex patterns that match will be removed from the document.action: action string to perform applying regex patterns, i.e.outputColName: output column name string which targets a column of type AnnotatorType.DOCUMENT.inpuColName: input column name string which targets a column of type Array(AnnotatorType.DOCUMENT).Let’s make a pass on the different parameters we are going to set in our example. +-+ | text| +-+ | ]*>"] replacement = " " removalPolicy = "pretty_all" encoding = "UTF-8" documentNormalizer = DocumentNormalizer() \. Let’s load some data to a text column in your input Spark SQL DataFrame: path = "html-docs" data = (path) df = data.toDF(schema=).select("text") df.show(). E.g., an ML model is a Transformer that transforms a DataFrame with features into a DataFrame with predictions. A Transformer is an algorithm that can transform one DataFrame into another DataFrame.
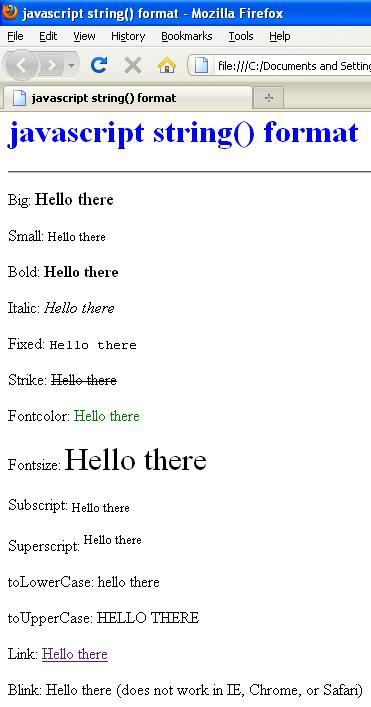
E.g., a learning algorithm is an Estimator that trains on a DataFrame and produces a model.

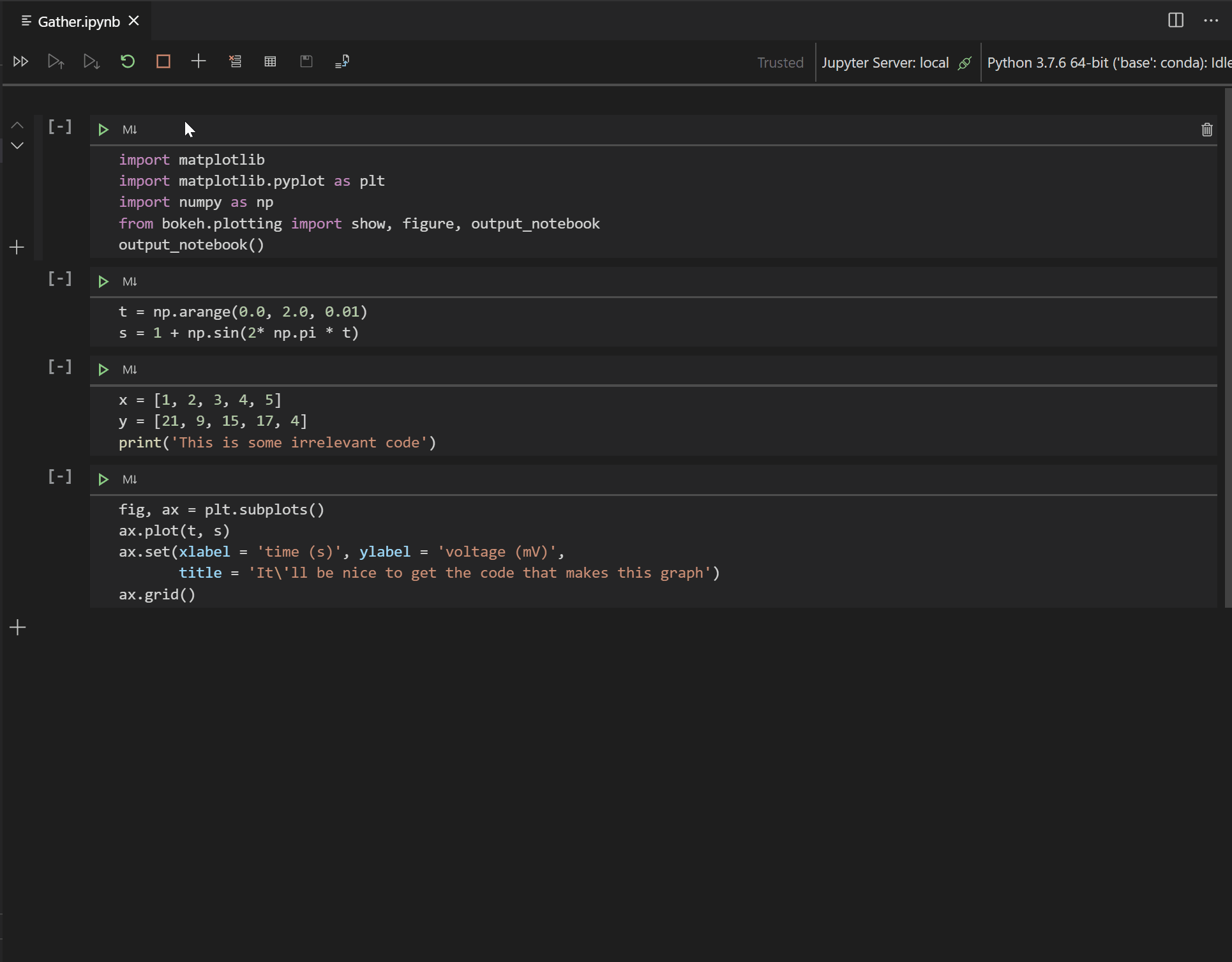
An Estimator in Spark ML is an algorithm that can be fit on a DataFrame to produce a Transformer. In Spark NLP, all Annotators are either Estimators or Transformers as we see in Spark ML. Please don’t call the ghostbusters, just use the brand new Spark NLP DocumentNormalizer annotator! :Dīut wait, what is an annotator? o.O Let’s see the definition to have an idea. Imagine you aggregate a collection of raw HTML documents you just collected from a given data source with your preferred crawler library and you want to remove all the tags to focus on the tag contents. Spark NLP community expressed the need for an annotator capable of directly processing input HTML/XML documents to clean or extract specific contents. Today I’m going to talk about a new annotator that was added in the latest release: the DocumentNormalizer. 720+ new pretrained models and pipelines while extending our support of multi-lingual models to 192+ languages such as Chinese, Japanese, Korean, Arabic, Persian, Urdu, and Hebrew.This includes new annotators for Google T5 (Text-To-Text Transfer Transformer) and MarianMNT for Neural Machine Translation - with over 646 new pre-trained models and pipelines. support to state-of-the-art Seq2Seq and Text2Text transformers.more accurate, faster, and support up to 375 languages.The writelines() method accepts an iterable object, not just a list, so you can pass a tuple of strings, a set of strings, etc., to the writelines() method.Some more impressive numbers from the latest 2.7.x release: The writelines() method write a list of strings to a file at once.The write() method writes a string to a text file.The open() function returns a file object that has two useful methods for writing text to the file: write() and writelines(). Open a text file for updating (both reading & writing). If the file exists, the function append contents at the end of the file. If the file doesn’t exist, the function creates a new file. If the file exists, the function will truncate all the contents as soon as you open it. The mode parameter specifies the mode for which you want to open the text file.įor writing to a text file, you use one of the following modes: Mode.The file parameter specifies the path to the text file that you want to open for writing.The open() function accepts many parameters.
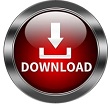